Teaching AI to See Our Best Side

We teach our machines every day. Voice assistants learn how to talk to us based on what we say to them. Navigation apps guide us based on the routes we take. Without millions of teachers like you, our machines would be much dumber.
But, for the most part, that relationship is passive. And that begs the question, what if we could teach our machines more actively? Could we school them on very specific things that are important to us? That thought became the perfect setup to build a little experiment involving selfies.
A self-portrait or a selfie is actually more than just one picture. It’s one picture out of endless tries which all look pretty much the same ... except that they don’t. At least not to the person taking the self-portrait. That person’s individual aesthetic, packed with subtle and subjective nuances, must be captured at exactly the right moment. Adding to the challenge, it's hard to explain to someone else the way you squint your eyes when you nail your smile or how you purse your lips to look roguish.
Enter Brainchild: a machine learning–based camera that can be taught individual preferences and make them accessible as a function. You can train the camera to understand how you like to see yourself without going through 100 iterations. And most importantly, it allows you to share your sensibility with somebody else.
Brainchild is just a prototype for now, but I'm hoping others will be inspired to build on the idea.

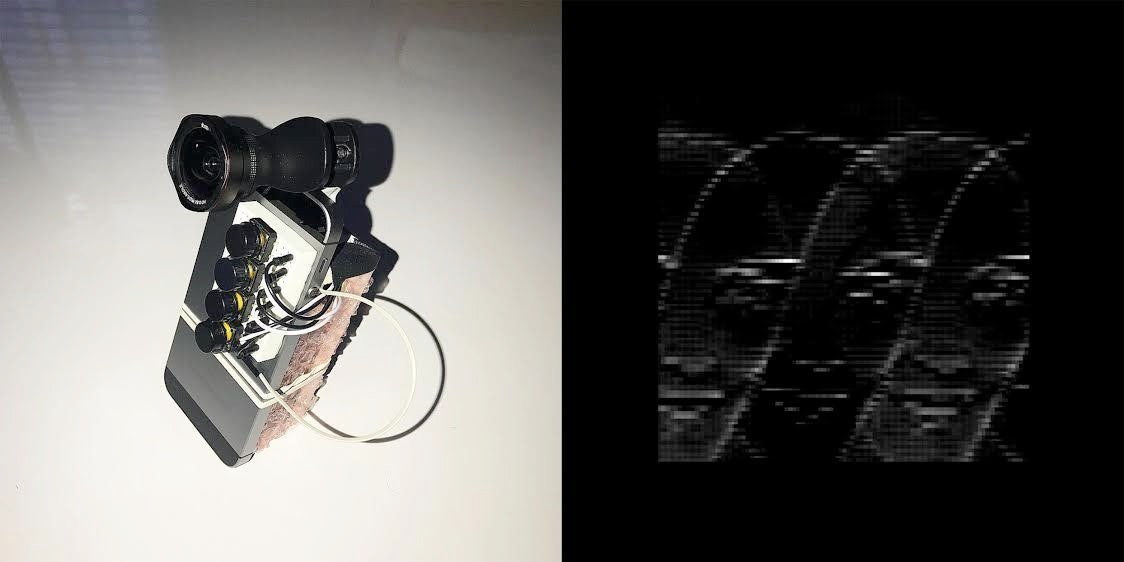
Beautiful, baby, beautiful
Machines are a billion times faster in quantitative tasks than we are. For a long time, the problem was one of not understanding quality. But that has changed with the improvement of so-called deep learning techniques—a subset of AI or machine learning.
By teaching Brainchild how you would like to be portrayed, it can assess the quality of what it sees in real time, and give you haptic feedback when it perceives you looking your best, so you can simply take that one picture.
It also allows others taking a snapshot of you to see you as if through your own eyes. Taking portraits is a very intimate art. Rather than taking the human out of the loop, Brainchild augments this human-to-human interaction by making it more collaborative.
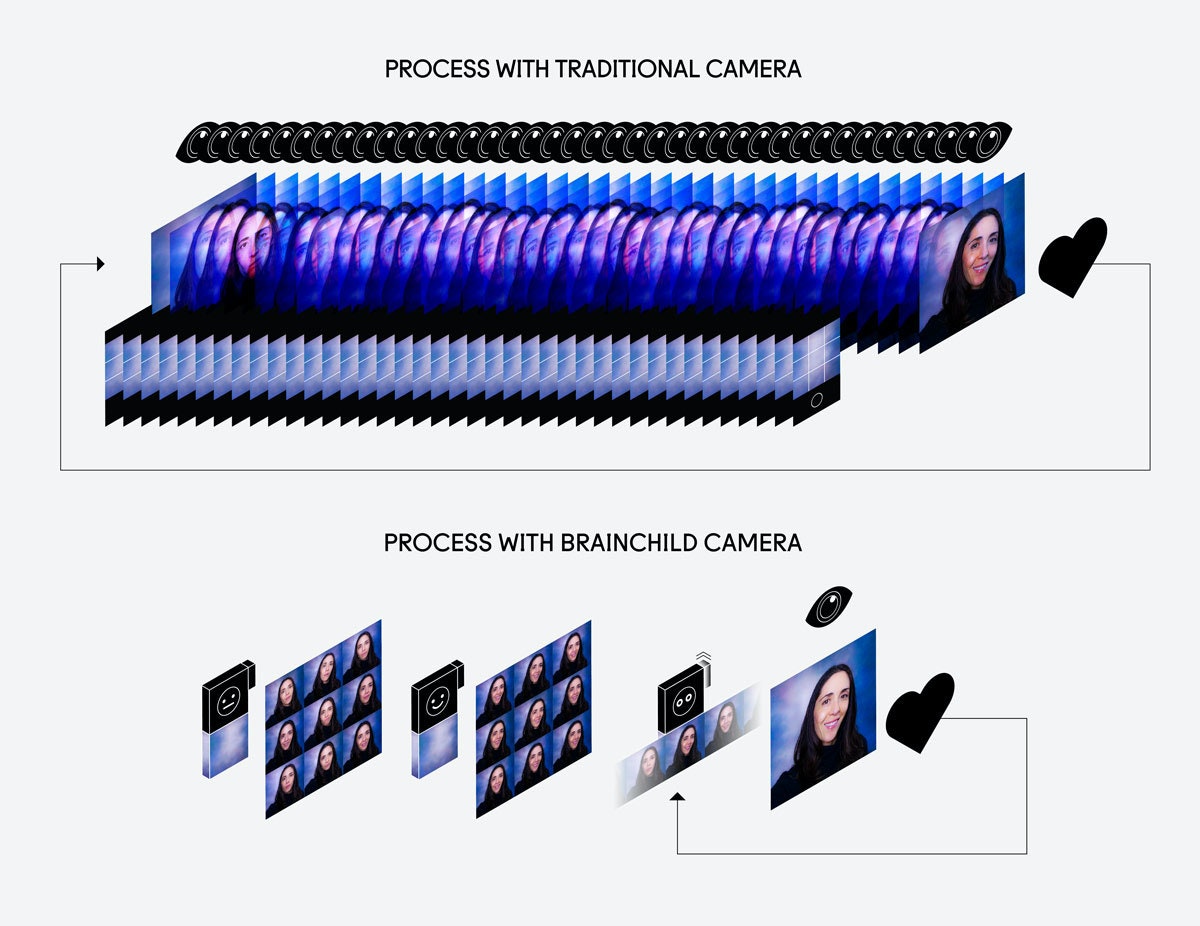
A feel-good feedback loop
The intervals between interacting with machine-learning products and experiencing their learnings are often very long. There are many technical challenges, but more accessibility and immediate feedback would help us teach more actively and develop a better intuition for them. In return, machines could learn better and become more personal.
Brainchild is designed to provide immediate feedback. You teach it how you like to be portrayed, instead of letting it guess, and every picture you take based on its haptic recommendation serves as feedback on how well it learned and how well you taught it. (Plus, it's private. Brainchild works offline and stores all of your information locally, so there's no danger of it being shared.)
Smile!
How does the Brainchild prototype work? There are countless technical parameters we could talk about, but in the most simple terms, it makes use of a so-called transfer learning process based on a fine-tuned convolutional neural network—a technique frequently used in computer vision.
Brainchild needs to be taught five fundamentals:
- What a human face looks like
- How to learn about new faces
- How to isolate the face in a picture
- The way the portrait subject looks normally
- The way the portrait subject looks when they deem themselves looking their best
The first two points require huge amounts of coding, data, number crunching, and time. That’s what consumed most of my time (next to working on the wrong file for a day!).
The third point addresses the fact that when you take a portrait there is always something behind you. To focus only on you without distraction, Brainchild extrudes your face from the background. That is something it learns before you use it.
Then you become the teacher. In order to learn how you look your best, it needs to know how you look normally. So, you feed it a few examples of both, either by taking new snapshots, or by using pictures stored on your phone.
To help Brainchild differentiate between the two picture sets you simply twist the front plate, switching between two learning modes. The serious smiley means every picture you take will teach Brainchild how you look “normally”. Switching to the happy smiley means every picture you take will teach it what it looks like when you look your best. This is how you get to know each other.
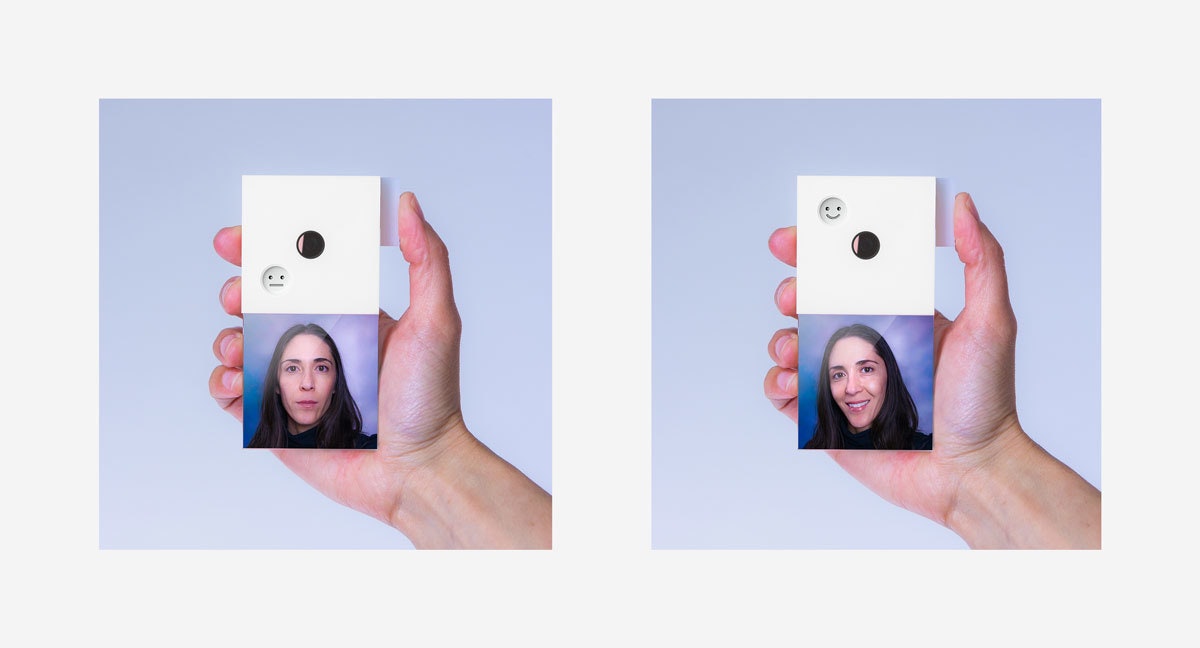
As soon as the camera spots you in a way that matches what you taught it about looking good, it will gently vibrate and light up the camera’s trigger button. The vibration and brightness increase the closer your match with your own ideal.
Brainchild doesn’t snap the photo for you, it just signals you at the optimal moment to do so. You can also hand the camera to someone else to capture you in the way you like to be captured. And the more you teach it, the more accurate it becomes.
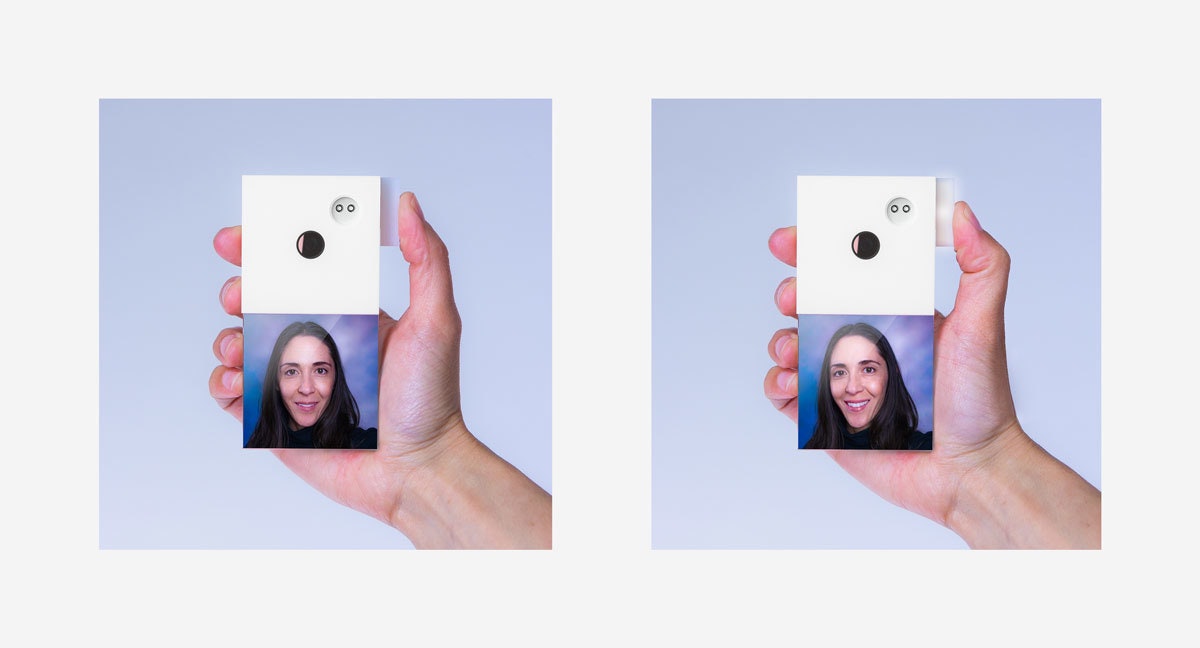
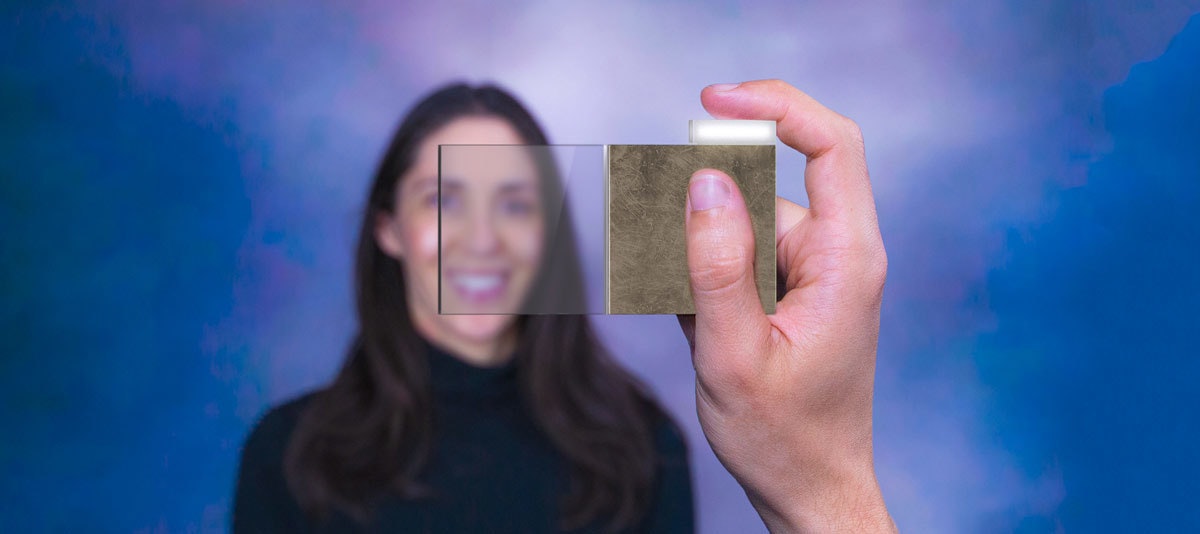
It might seem mundane to teach a camera how to take a better selfie, but the technology has already inspired one of our clients to rethink parts of a high precision medical device that could dramatically reduce treatment costs for patients, and another software engineer to work on a playful version of FaceID.
I myself am now working on a body pose add-on for fashion photography, and am curious to see all of the other ways we might actively teach our machines and help them augment us in more personal ways.
Instagram: @brain_children
Concept and Technology: Jochen Maria Weber
Visual Design: Tiffany Yuan
Industrial Design: Leo Marzolf
Special thanks: Tobias Toft



Get in touch